We’ve been seeing a lot of our customers implementing ML Flow as a part of their MLOps roadmap, they tend to want something more for computer vision, and that’s when Picsellia comes in.
While MLFlow is great for managing the entire classical machine learning model lifecycle with its strong experiment tracking, model management, and deployment capabilities, it doesn't quite meet the specific needs of computer vision.
That's where Picsellia comes in, filling these gaps with its tailored MLOps tools for computer vision, ranging from visual data annotation, centralized data management, and advanced visualization to production data & model monitoring. By combining MLFlow’s comprehensive ML lifecycle management with Picsellia’s specialized features for computer vision, you can create a workflow that is streamlined, efficient, and scalable.
This integration ensures you have high-quality data, better-performing models, and smooth deployment, speeding up the development and deployment of robust computer vision solutions.
If you want to jump into our how-to guide → here you go
Why using MLFlow?

MLFlow is a central tool for your entire machine-learning lifecycle. It logs and organizes all the parameters, metrics, and artifacts from experiments, ensuring everything is reproducible. Moreover, MLFlow provides a centralized model registry, making it easy to version and deploy models efficiently, which helps maintain consistency and reliability across different projects.
The platform also simplifies the packaging and deployment of models across various environments, making the transition from development to production smooth and straightforward. Its compatibility with popular ML libraries and platforms allows it to integrate seamlessly into existing workflows, boosting productivity and collaboration. Additionally, MLFlow's scalability supports large-scale machine learning projects, fostering continuous improvement and innovation.
However, while MLFlow is excellent for managing data science projects, it has its limitations when it comes to computer vision. To fix these limitations, integrating complementary tools becomes necessary.
Limitations of MLFlow for Computer Vision
Despite its many strengths, MLFlow has some drawbacks when it comes to computer vision projects. It lacks specialized tools for handling and preprocessing visual data, which are crucial for VisionAI. As a computer vision scientist, you sure know that you definitely can’t handle a CV project like any tabular data project, you need visualization at every step.
Furthermore, MLFlow does not offer built-in annotation tools, making it difficult to create and manage labeled datasets necessary for training models. Handling large volumes of image data and artifacts can cause performance bottlenecks, underlining scalability issues.
Additionally, MLFlow’s basic visualization capabilities fall short for tasks like displaying image transformations or segmentation results.
These limitations highlight the need for complementary tools to achieve a comprehensive computer vision workflow.
That’s when Picsellia comes in.
How Picsellia Complements MLFlow for Computer Vision
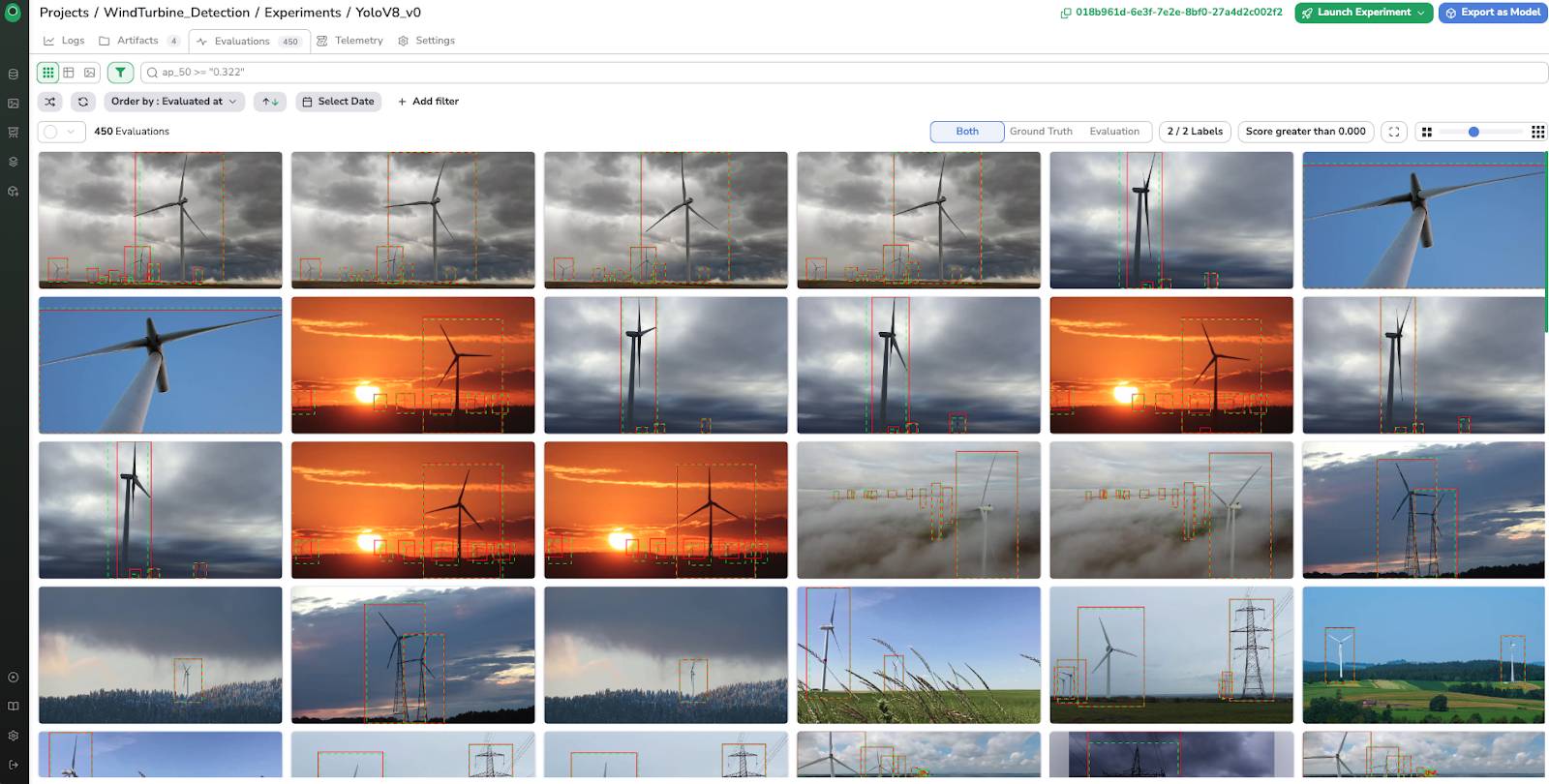
Picsellia addresses MLFlow's limitations by providing specialized tools and features necessary for computer vision. The platform was created to handle large volumes of visual data, offering efficient tools for preprocessing and data management, which are crucial for computer vision projects.
Picsellia's comprehensive annotation tools facilitate the creation and management of labeled datasets, streamlining the training process. Designed to handle extensive computer vision projects, Picsellia ensures smooth performance even with large datasets, overcoming scalability challenges. Its seamless integration with MLFlow and other MLOps frameworks simplifies and streamlines workflows, enhancing productivity. Additionally, Picsellia offers advanced visualization tools for both annotation and predictions allowing computer vision engineers to understand how their models behave.

Conclusion
Integrating Picsellia with MLFlow creates a powerful synergy for managing computer vision projects. MLFlow provides robust experiment tracking, model management, and deployment capabilities across the whole data science spectrum (structured and unstructured data). However, it’s barely impossible to fit 100% of the needs of Computer vision engineers with a generalist platform. That’s why Picsellia exists.
The combination of both platforms ensures that your Data Science organizations still share the same baseline in terms of MLOps, while providing the right tools for Computer Vision requirements.